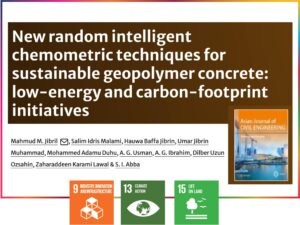
Researchers from Near East University have contributed to a study addressing the environmental impact of the construction industry by exploring sustainable alternatives to traditional Portland cement binders. Given the industry’s significant contribution to greenhouse gas emissions, the study focuses on geopolymer as a promising and eco-friendly substitute. Geopolymer offers a low-energy, low-carbon-footprint solution for various civil infrastructure applications.
The research evaluates five predictive models—random forest (RF), robust linear regression (RL), recurrent neural network (RNN), response surface methodology (RSM), and regression tree (RT)—to forecast the compressive strength (CS) of Geopolymer Concrete (GPC). RL and RT models were implemented using Matlab R19a regression learner APP, while RNN was executed with the Matlab R19a toolkit. RF development employed R studio version 4.2.2 programming code, and the RSM model was constructed using the Minitab 18 toolbox. EViews 12 software facilitated data pre-processing, post-processing, and conversion of non-stationary data into stationary data for accurate results.
The study considers various input variables, including SiO2/Na2O (S/N), Na2O (N), water/binder ratio (W/B), curing time (CT), ultrasonic pulse velocity (UPV), and 28-day compressive strength (MPa) (CS) as the target variable. Notably, the RSM-M3 model outperformed other models, demonstrating superior accuracy with a Pearson correlation coefficient (PCC) of 0.994 and a mean absolute percentage error (MAPE) of 0.708 during the verification phase.
Given that compressive strength is a critical mechanical characteristic in concrete, accurately predicting it is essential to prevent severe failures and facilitate effective repair. GPC’s complexity makes predicting its behavior challenging, prompting the exploration of various models. The study concludes that the proposed models, particularly the RSM-M3, offer valuable tools for predicting the compressive strength of GPC, highlighting their potential for enhancing sustainable practices in the construction industry.
This research contributes to advancing sustainable construction practices and demonstrates the effectiveness of geopolymer as an environmentally friendly alternative to traditional cement binders, aligning with global efforts to mitigate the environmental impact of construction activities.
More Information:
https://link.springer.com/article/10.1007/s42107-023-00908-7
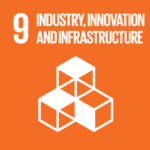
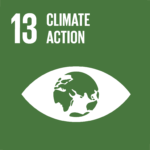
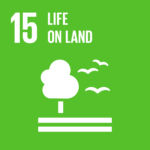