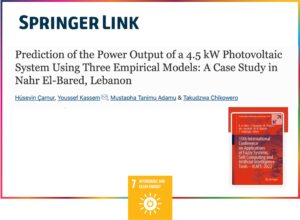
Researchers from the Faculty of Engineering at Near East University, including Çamur, Kassem, Adamu, and Chikowero, have conducted a study focusing on predicting electricity production (EP) from a 4.5 kW photovoltaic (PV) system in Nahr El-Bared, Lebanon. The study utilizes Multilayer Feed-Forward Neural Network (MFFNN) and Cascade Feed-forward Neural Network (CFNN) models, comparing their accuracy with the response surface methodology (RSM).
Hourly data of beam irradiance (BI), diffuse irradiance (DI), reflected irradiance (RI), sun height (SH), air temperature (AT), and wind speed (W) were collected and utilized as input parameters for the proposed models. The results indicate that all proposed models effectively predict the EP of the PV system. However, among the developed models, the CFNN model demonstrates significantly better prediction performance based on the metrics of R2 and RMSE.
This research provides valuable insights into the effectiveness of neural network models in predicting electricity production from PV systems, offering a promising approach for optimizing energy generation in solar installations. By accurately forecasting EP, stakeholders can make informed decisions regarding system design, operation, and maintenance, ultimately enhancing the efficiency and sustainability of solar energy utilization.
Furthermore, the study underscores the importance of leveraging advanced computational techniques, such as neural networks, in renewable energy research. Such methodologies enable researchers to analyze complex datasets and develop robust predictive models, contributing to the advancement of renewable energy technologies.
Overall, the findings of this study contribute to the body of knowledge in the field of solar energy forecasting and demonstrate the potential of neural network models in improving the performance of PV systems. The research conducted by the Near East University’s Faculty of Engineering offers valuable insights for the optimization of solar energy utilization, paving the way for more efficient and sustainable energy solutions.
More Information:
https://link.springer.com/chapter/10.1007/978-3-031-25252-5_32
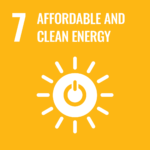