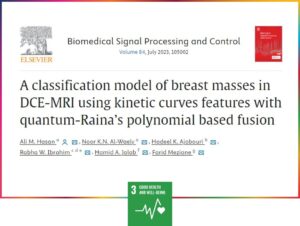
A collaborative study, including a researcher İbrahim from Department of Mathematics, Near East University, has introduced an approach to improve the classification accuracy of breast lesions in Dynamic Contrast Enhanced MRI (DCE-MRI). Breast cancer, the most prevalent cancer among women globally, necessitates early detection for effective treatment. While DCE-MRI offers superior insights into tumor morphology and microenvironment, previous research often overlooked essential breast cancer characteristics crucial for accurate identification and differentiation from benign pathologies.
The study proposes a novel fusion model that integrates kinetic curves features with a newly devised Quantum-Raina’s Polynomial (QRP) feature extraction method. By combining these features, the classification accuracy of breast lesions into benign and malignant categories was significantly enhanced, achieving a remarkable 97.4% accuracy rate on a dataset comprising 300 DCE-MRI scans.
The fusion of kinetic and QRP features enables a quantitative differentiation between benign and malignant breast lesions, offering a substantial improvement over existing classification techniques. Notably, the proposed model demonstrates a low complexity rate, making it both efficient and effective in clinical settings.
Key to the study’s innovation is the integration of kinetic and QRP features (TIC-QRP), which outperforms conventional methods in breast mass classification. However, the manual assessment of Regions of Interest (ROI) and TIC analysis by radiologists poses a limitation due to microscopic alterations within tumors, affecting ROI detection accuracy.
To address this limitation, future research should focus on automating ROI delineation and TIC analysis. Additionally, efforts to identify specific histopathological and molecular subtypes of breast cancer based on DCE-MRI data would further enhance diagnostic accuracy.
Furthermore, extending post-contrast delayed phase imaging to at least 5 minutes post-injection is recommended for efficient differentiation between benign and malignant breast lesions. Future endeavors should also consider utilizing larger datasets of publicly available DCE-MRI scans to validate and refine the proposed model.
Overall, this study represents a significant advancement in breast cancer diagnosis through DCE-MRI, offering a promising avenue for improving clinical outcomes and patient care.
More Information:
https://www.sciencedirect.com/science/article/pii/S1746809423004354
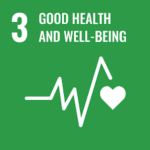