In a collaborative study involving a researcher from Near East University, an innovative approach to optimizing the Industrial Internet of Things (IIoTs) networks has been developed. This research presents an optimized bio-inspired localization routing technique, which is crucial for the sustainability of IIoT networks and the advancement of green cities.
The IIoT network, essential in modern industrial applications, often faces challenges due to the energy limitations and computational capabilities of sensor nodes (SNs). Addressing this, the study introduces an improved evolutionary algorithm called Cat Swarm Optimization (CSO) to enhance node localization and cluster-based routing, thereby reducing energy consumption in IIoT networks.
This approach is significant in two aspects. Firstly, it uses the CSO method for optimizing the bio-inspired node’s location, which is pivotal in minimizing localization errors. Secondly, it employs a cluster-based routing technique that conserves energy in SNs by minimizing the average distance between the cluster and its SNs while selecting energy-efficient Cluster Heads (CH).
Comparative analysis with traditional methods like Particle Swarm Optimization (PSO) indicates that the Improved CSO (ICSO) algorithm demonstrates superior performance in terms of convergence rate, network lifetime, and fitness value. These improvements are vital for achieving sustainable IIoT networks, which are integral to the development of smart, green cities.
Furthermore, the study explores the real-time application of these algorithms, assessing their performance in node localization and energy-efficient clustering. The results showcase that the ICSO algorithm not only outperforms the PSO in localization error and computing time but also shows resilience against environmental factors like additive Gaussian noise and beacon density.
Looking forward, the study suggests the potential of combining the ICSO algorithm with other evolutionary algorithms to further reduce location estimation errors, contributing to the efficiency of IIoT networks. The implications of this research extend beyond industrial applications, supporting the broader goals of sustainable urban development, efficient resource management, and innovation in city infrastructure.
In summary, the research involving Near East University represents a significant advancement in IIoT networks, offering a more sustainable, energy-efficient solution that aligns with the objectives of developing smart and eco-friendly urban environments.
For further details, access the original paper from the publisher’s link:
https://www.sciencedirect.com/science/article/pii/S2210670723003335
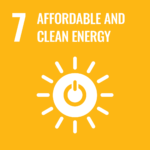
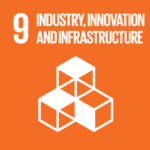
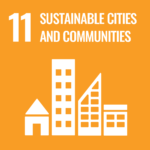