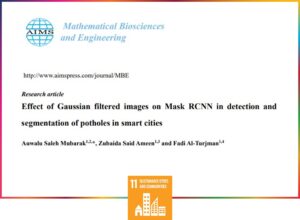
A collaborative research effort led by researcher Mubarak from Artificial Intelligence Engineering Dept., AI and Robotics Institute, Near East University, along with co-authors from other institutions, has tackled the critical issue of road safety by developing an efficient model for pothole detection on vehicles. Potholes are a leading cause of accidents worldwide, emphasizing the urgent need for reliable detection systems.
The study focuses on creating a lightweight yet accurate model suitable for real-time detection, particularly on edge and mobile devices. Leveraging deep learning and computer vision techniques, the team employed a Mask RCNN model with ResNet-50 and MobileNetv1 as backbone architectures to enhance detection capabilities.
A notable aspect of the study involved comparing the model’s performance using original training images versus images filtered with a Gaussian smoothing filter. Remarkably, the results demonstrated that training the ResNet-50 with Gaussian filtered images significantly outperformed other models evaluated in the study. This finding underscores the effectiveness of Gaussian filtering in enhancing model performance by reducing noise and improving object visibility, crucial for accurate detection.
The experimentation with different backbone architectures revealed promising results in pothole detection and segmentation. Although both ResNet-50 and MobileNetv1 exhibited satisfactory performance levels, the study suggests that utilizing larger datasets could further enhance model performance.
Looking ahead, the researchers plan to explore additional backbone architectures and improved structures to bolster performance further. By continuously refining and optimizing the model, they aim to develop even more robust and reliable pothole detection systems for enhanced road safety.
In summary, this collaborative effort represents a significant advancement in the field of road safety, offering a practical solution for detecting potholes on vehicles in real-time. The findings hold promise for the development of more effective detection systems, ultimately contributing to the prevention of accidents and preservation of lives and property on roads worldwide.
More Information:
https://www.aimspress.com/aimspress-data/mbe/2023/1/PDF/mbe-20-01-013.pdf
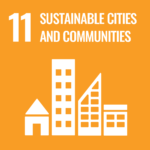