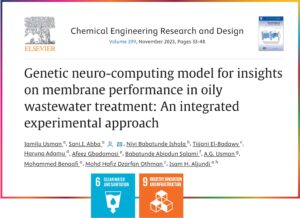
Researcher from Near East University collaborated on a study focused on sustainable water treatment, aligning with the United Nations’ Sustainable Development Goal 6 (SDG 6): Clean Water and Sanitation. The study employed advanced modeling techniques, combining Response Surface Methodology (RSM) and an Artificial Neural Network-based Genetic Algorithm (ANN-GA), to predict key parameters influencing membrane performance in the context of oil separation.
The central composite design (CCD) screening approach of RSM was applied to assess the impact of crucial process input parameters, including oil concentration, feed flow rate, and feed pH, on separation efficiency and oil flux. Both the RSM model and ANN-GA were adeptly utilized to identify optimal conditions for maximizing membrane performance.
Statistical analyses, employing determination coefficients (R²), standard error of prediction (SEP), analysis of variance (ANOVA), and t-tests, demonstrated the precision of both models in describing the membrane performance process. For oil flux, the RSM model yielded an estimated R² of 0.9916 and SEP of 3.54%, while the ANN model exhibited an R² of 0.9933 and SEP of 3.31%. In terms of separation efficiency, the RSM model achieved R² = 0.9929 and SEP = 1.31%, whereas the ANN model excelled with R² = 0.9961 and SEP = 0.99%. Notably, the ANN-GA approach identified the most favorable conditions for both responses.
The study emphasized the significance of the oil concentration, feed pH, and feed flow rate, with oil concentration proving to be the most influential factor. This research contributes to the implementation of sustainable monitoring and management practices in various industries, aligning with SDG 6’s aim to ensure the availability and sustainable management of water and sanitation for all.
Looking ahead, the study advocates for the broader application of machine learning in addressing challenges in oil separation within the oil and gas industry. Future research directions include scaling up the membrane treatment process to industrial levels, considering factors such as membrane fouling and system maintenance. Comprehensive studies involving a broader set of input variables are also recommended to enhance model accuracy and applicability in real-world scenarios.
More Information:
https://www.sciencedirect.com/science/article/pii/S0263876223005919?via%3Dihub
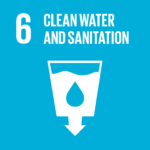
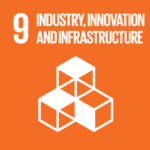