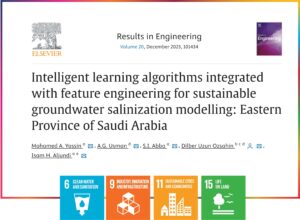
A collaborative study by researchers at Near East University addresses the critical issue of groundwater (GW) salinization, a process where GW accumulates higher salt content due to both natural and anthropogenic factors. The research, featuring co-authors from Near East University, employs advanced feature extraction and machine learning (ML) techniques, including Gaussian Process Regression (GPR), Support Vector Regression (SVR), Regression Tree (RT), and Robust Linear Regression (RLR), to assess and model the salinization phenomenon.
Groundwater salinization can result from various factors such as geological influences, proximity to the ocean, irrigation practices, land use changes, and industrial or municipal waste discharge. The research focuses on developing robust models to predict salinity levels, crucial for managing water resources, particularly in regions facing water scarcity and quality challenges.
The study showcases the efficiency of ML algorithms, with GPR-Comb-3 emerging as the top-performing model in both training and validation stages, exhibiting high precision correlation coefficients. Notably, even the classical RLR model demonstrates exceptional prediction skills, emphasizing the significance of combining traditional and AI-enhanced methods.
Furthermore, the researchers introduce the innovative integration of sensitivity analysis techniques, namely NFSA (Normalized Forward Sensitivity Analysis) and LCA (Local Contribution Analysis), enhancing prediction accuracy for all model combinations. This integration stands as a novel approach, contributing to the existing body of knowledge on GW salinization modeling.
In addition to quantitative parameters, the study introduces a Water Quality Index (WQI) incorporating physical, chemical, and biological factors, providing a comprehensive evaluation of Total Dissolved Solids (TDS) in Saudi Arabian GW. The models, trained on parameters such as Total Hardness, Na+, Mg2+, Ca2+, F−, Cl−, Fe, and EC, present a holistic approach to water quality assessment.
The research underlines the importance of integrating ML models with cutting-edge techniques for better GW salinization prediction. The results contribute to Sustainable Development Goals (SDGs) 6 (Clean Water and Sanitation), 9 (Industry, Innovation, and Infrastructure), 11 (Sustainable Cities and Communities), and 15 (Life on Land), emphasizing the need for informed policies to manage and mitigate environmental challenges associated with water resources.
The future scope of the study suggests further enhancements through the integration of ML with remote sensing and the Internet of Things (IoT) for comprehensive monitoring of GW salinity. Such advancements can provide valuable insights into spatial and temporal variations in salinization, aiding policymakers and government agencies in sustainable water resource management.
More Information:
https://www.sciencedirect.com/science/article/pii/S2590123023005613
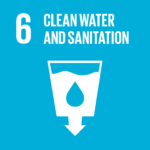
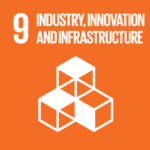
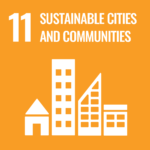
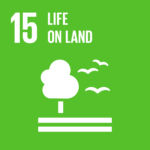