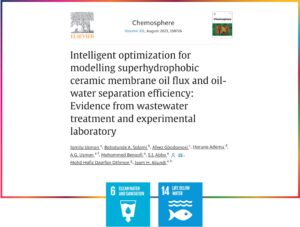
A collaborative research effort, including a co-author Usman from Faculty of Pharmacy, Near East University, has advanced the field of oil-water separation through the application of advanced AI-based optimization techniques. With the aim of improving the efficiency of membrane-based separation processes, the study focused on predicting oil flux and oil-water separation efficiency using support vector regression (SVR) and Gaussian process regression (GPR) models, augmented by the Response Surface Method (RSM).
By considering input variables such as oil concentration, feed flow rate, and pH, the study developed various combinations to discern the most influential factors affecting model performance. Evaluation metrics including mean square error (MSE) and Nash-Sutcliffe coefficient efficiency (NSE) were employed to gauge prediction accuracy across different input combinations.
Results indicated that SVR outperformed GPR in predicting oil flux, with the SVR-2 [Combo-2] model exhibiting higher accuracy. Conversely, GPR demonstrated superior performance in predicting oil-water separation efficiency, particularly with the GPR-3 [Combo-3] model. These findings underscore the versatility of machine learning techniques in addressing complex separation phenomena.
Furthermore, the study’s outcomes have significant implications for both academia and industry. Academically, the adoption of machine learning strategies enhances understanding and governance of membrane performance features, facilitating advancements in areas such as oil flux prediction, separation efficiency, water recovery, and membrane fouling. For manufacturers and practitioners, these insights enable optimization of design and operation processes, leading to increased productivity, cost reduction, and improved environmental sustainability.
The research underscores the importance of AI-based optimization in addressing challenges within the oil and gas industry, particularly in developing sustainable and eco-friendly separation technologies. By harnessing the predictive power of machine learning models, policymakers can make informed decisions to enhance environmental performance and resource utilization.
This collaborative study showcases the efficacy of combining advanced machine learning techniques with classical approaches to advance oil-water separation processes. The robust performance of SVR and GPR models underscores their potential in solving complex separation phenomena, ultimately contributing to enhanced efficiency and sustainability in the oil and gas sector.
More Information:
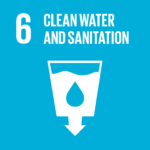
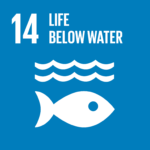