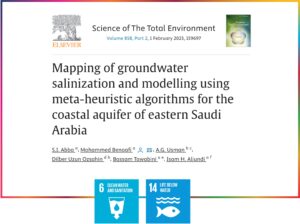
A collaborative effort between researchers from Saudi Arabia and Usman and Özşahin from Near East University has yielded a significant study addressing the escalating issue of groundwater salinization in coastal aquifers. This research, aimed at enhancing water resource planning and management, employed a multifaceted approach combining field investigations, experimental laboratory analyses, and artificial intelligence (AI)-based optimization techniques.
By integrating real-world data with advanced computational methods, namely adaptive neuro-fuzzy inference system (ANFIS) hybridized with genetic algorithm (GA), particle swarm optimization (PSO), and biogeography-based optimization (BBO), the study accurately identified and predicted groundwater salinization levels in the eastern coastal region of Saudi Arabia. Utilizing ArcGIS 10.3 software, prediction maps were generated based on the optimized AI models, offering valuable insights into spatial distribution patterns.
Feature selection, facilitated by the PSO algorithm, identified key parameters influencing groundwater salinity, with Ca2+, Na+, Mg2+, and Cl− emerging as the most influential factors. Through rigorous statistical evaluation, the ANFIS-PSO algorithm demonstrated the highest accuracy (99%) among the models tested, followed by ANFIS-GA and ANFIS-BBO.
The study’s findings underscore the efficacy of integrating traditional hydrochemical analyses with AI-driven optimization techniques in addressing complex water management challenges. Notably, the research highlights the importance of Na+ as a dominant input variable in assessing salinity concentrations, corroborated by survey results indicating its significance.
In addition to providing valuable insights for policymakers and water resource management agencies, the study emphasizes the need for continued exploration of alternative machine learning approaches to further refine salinity estimation models in coastal aquifers. By leveraging a combination of experimental analysis, computational algorithms, and spatial-temporal assessments, the study offers a comprehensive framework for mitigating groundwater depletion and safeguarding vital water resources in Saudi Arabia’s coastal regions.
This collaborative endeavor represents a significant step towards addressing the pressing issue of groundwater salinization, aligning with the sustainable development goals outlined in Vision 2030. Through interdisciplinary collaboration and innovative methodologies, the study sets a precedent for evidence-based decision-making in water resource management, with far-reaching implications for arid regions facing similar challenges worldwide.
More Information:
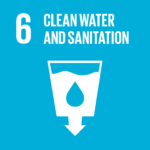
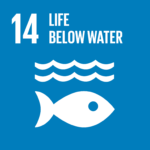