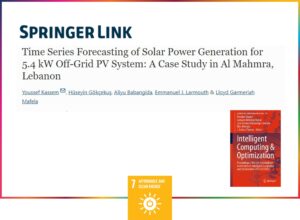
A study led by researchers Kassem, Gökçekuş, Babangida, Larmouth and Mafela from Near East University presents innovative models for predicting power production (PP) from an off-grid photovoltaic (PV) system in Al Mahmra, Lebanon. The research focuses on developing and employing Multi-Layer Perceptron Neural Network (MLPNN), Quadratic model (QM), and Multiple Linear Regression (MLR) to forecast PP accurately.
Hourly data of wind speed (WS), global solar radiation (GSR), average temperature (T), and time (Ti) are utilized as input parameters for the models. Through rigorous analysis, the study demonstrates the effectiveness of QM and MLPNN models in predicting PP from the PV system. Among the models developed, the Quadratic model stands out for its superior prediction performance, as evidenced by key metrics such as R2, MAE, and RMSE.
The findings underscore the importance of employing advanced modeling techniques to enhance the efficiency and reliability of power production prediction from renewable energy sources like PV systems. By leveraging machine learning algorithms and mathematical models, researchers can accurately forecast PP, enabling better resource management and decision-making in energy systems.
The study highlights the potential of MLPNN, QM, and MLR models in predicting power production from off-grid PV systems. The superior performance of the Quadratic model demonstrates its suitability for accurate PP forecasting. These insights contribute to advancing the understanding and application of predictive modeling in renewable energy systems, paving the way for more efficient and sustainable energy production practices.
More Information:
https://link.springer.com/chapter/10.1007/978-3-031-19958-5_58
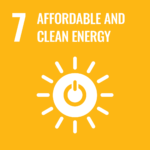